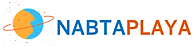
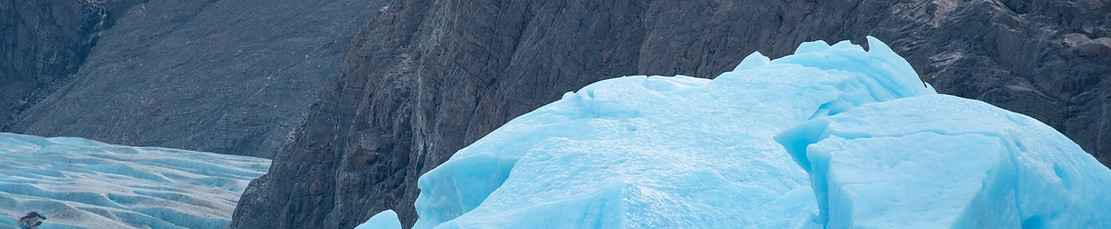
Research Projects
Agriculture
Precision agriculture is a cutting-edge approach to farming that utilizes advanced technologies and data-driven techniques to optimize productivity and efficiency while minimizing environmental impact. In our project with students from Beni Suef University we developed algorithms to prototype an End-to-End solution from gathering satellite data to finally providing maps for different agriculture use cases. By gathering the data and analyzing it using image processing, deep learning and GIS techniques, a wide range of Agricultural information/products can be provided to serve a broad spectrum of users like farmers, researchers and decision makers. That results in reducing costs, increase crop production enhanced crop yields, making precision agriculture a promising solution for sustainable and efficient food production in the face of increasing global challenges. In this cooperation, we successfully provided feasibility studies for crop classification and crop diseases.
Crop Classification
In crop classification, we use a combination of optical and radar satellites like sentinel-2 and sentinel-1 to train our AI model in order to produce crop maps like for rice, corn, cotton, etc at 20 m resolution. The training data is created by the reference dataset provided by American Center for Spatial Information Science and Systems. The reference crop maps used in building our AI model is only available in USA. However, the models built can be also tested and validated over other areas in the globe if reference data is available. For example, a recently released crop type map model by ESA Cereal Model could be used for validation. Most of crop classification work was done for corn crops specifically in IOWA state in the USA (See Figure 1). An accuracy of 83% was reached. The model wasn’t including the temporal factor via using time series, but this will be our kept for future work.
Crop Diseases
While for crop diseases, Aerial imagery collected by drones were used. The training dataset contained information about crop diseases/issues like Weed cluster, Dry down, Nutrient deficiency and Endrow. The dataset is a public one and provided by Vision for Agriculture that host AI challenges. Each image consists of four 512x512 (10 cm) color channels, which are RGB and Near Infra-red (NIR). Each image also has a boundary map and a mask for the related problem. In order to reduce the computation power needed, the images were scaled to 256x256 (20 cm) resolution. Several AI models with different architecture were experiment on the data. Different results/performance due to different models and different crop issues were analyzed (See Figure 3).

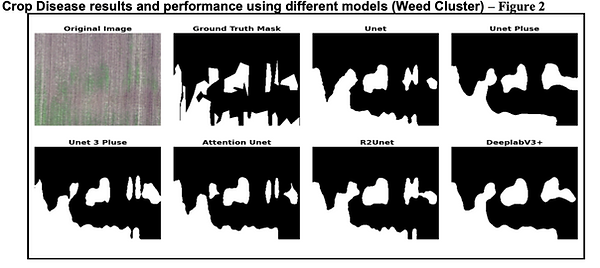
Crop classification
Crop Anomalies detection